How AI and Machine Learning Are Transforming Cybersecurity Quality Assurance
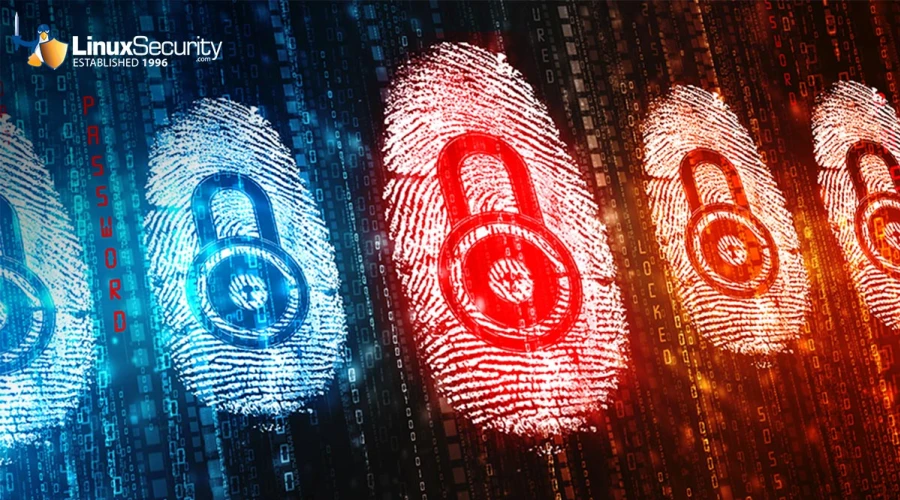
The landscape of cyber threats and Linux vulnerabilities is evolving at an unprecedented pace, making it imperative for organizations to adopt advanced security measures. While effective to an extent, traditional quality assurance (QA) methods may no longer suffice against sophisticated cyberattacks.
In this context, integrating Artificial Intelligence (AI) and Machine Learning (ML) into QA practices has emerged as a powerful solution. These technologies offer new ways to identify and mitigate vulnerabilities, ensuring a more robust and adaptive Linux security framework.
Quality assurance in cybersecurity traditionally involves systematic testing to ensure systems are secure and free from vulnerabilities. However, with the advent of AI and Machine Learning, QA practices are undergoing a significant transformation. These technologies enable real-time monitoring, predictive analytics, and automated threat detection, enhancing the effectiveness and efficiency of QA processes. In this article, we'll explore how AI and ML are reshaping QA in cybersecurity, the key practices involved, and the benefits they offer Linux admins and organizations.
Key Takeaways:
- AI and Machine Learning enhance the accuracy and efficiency of cybersecurity QA.
- They provide real-time monitoring, automated responses, and help ensure compliance.
- Key implementation steps include assessing current processes, selecting the right tools, and cross-functional collaboration.
- Challenges include data quality, algorithm biases, and the need for expertise, but best practices can help overcome these obstacles.
What Is The Role of Quality Assurance in Cybersecurity?
Quality assurance has always been a cornerstone of robust cybersecurity strategies. It involves a series of checks and balances to ensure that systems and applications are secure from potential threats. Traditionally, QA practices have focused on manual code reviews, penetration testing, and compliance checks. While effective, these methods can be time-consuming and prone to human error. Moreover, more than they may be required to counter increasingly complex cyber threats.
This is where AI and Machine Learning come into play. These technologies bring a new dimension to QA by automating many of the processes that were previously manual. AI-driven tools can quickly analyze vast amounts of data, identifying patterns and anomalies that may indicate a security threat. Machine Learning algorithms can learn from previous incidents, improving accuracy and efficiency. This speeds up the QA process and enhances its effectiveness, making it possible to identify and address vulnerabilities before they can be exploited.
Integrating AI and Machine Learning into QA practices significantly shifts how organizations approach cybersecurity. These technologies enable a more proactive and dynamic defense strategy, allowing real-time threat detection and response. As cyber threats continue to evolve, the role of AI and ML in QA will only become more critical.
Essential AI and Machine Learning Practices in QA
Implementing thorough QA protocols with AI-powered tools is a game-changer in cybersecurity. One of the most significant benefits of AI and Machine Learning is their ability to conduct security audits with unparalleled speed and accuracy. Traditional audits can be labor-intensive and slow, often leaving gaps in coverage. AI tools, on the other hand, can continuously scan and assess systems for vulnerabilities, providing real-time insights and alerts.
Anomaly detection is another key area where AI shines. Machine Learning algorithms can analyze normal system behavior and identify deviations that may indicate a potential threat. This is particularly useful for detecting insider threats or sophisticated attacks that might evade traditional detection methods. Predictive analytics, powered by Machine Learning, can also forecast potential security issues based on historical data and current trends, enabling organizations to take preventive measures.
The role of AI in automated threat detection and response cannot be overstated. Machine Learning models can be trained to recognize cyberattacks, such as phishing attempts or malware infections. Once detected, AI systems can automatically initiate responses, such as isolating affected systems or blocking malicious IP addresses. This level of automation speeds up the response time and reduces the likelihood of human error.
Continuous learning and adaptation are critical components of AI and Machine Learning systems. As these technologies encounter new threats, they can adjust their algorithms and improve their accuracy. This makes AI and ML invaluable assets in the ever-changing landscape of cybersecurity.
What Are the Benefits of Integrating AI and Machine Learning in QA?
Integrating AI and Machine Learning into quality assurance offers numerous benefits, enhancing the security and efficiency of cybersecurity measures. One of the most notable advantages is the improved accuracy and efficiency in identifying vulnerabilities. Traditional methods can be slow and may miss subtle signs of security issues. In contrast, AI-powered systems can analyze large datasets quickly, identifying patterns that might indicate a threat. This leads to a more comprehensive and accurate assessment of potential vulnerabilities.
Another significant benefit is real-time monitoring and automated responses. AI systems can continuously monitor network traffic and system behavior, detecting anomalies as they occur. This real-time capability allows for immediate action, such as isolating a compromised system or blocking malicious activities, minimizing the impact of potential threats. Automated responses also reduce the workload on security teams, allowing them to focus on more complex issues.
AI and Machine Learning also play a crucial role in ensuring compliance and regulatory adherence. Many industries are subject to stringent regulations regarding data security and privacy. AI tools can help organizations monitor compliance in real-time, ensuring that all systems and processes meet the required standards. This helps avoid legal penalties and builds trust with customers and stakeholders.
Furthermore, integrating AI and ML in QA enhances an organization's reputation and fosters customer trust. In a world where data breaches are increasingly common, a commitment to advanced security measures can be a significant differentiator. Customers are more likely to trust businesses that invest in cutting-edge technologies to protect their data.
What Are the Steps to Implement AI and Machine Learning in Cybersecurity QA?
Integrating AI and Machine Learning into your cybersecurity quality assurance strategy requires careful planning and execution. The first step is to assess your current QA processes and identify areas where AI can add the most value. This might involve evaluating the effectiveness of existing security measures and determining the level of automation that can be achieved.
Next, selecting the right AI and Machine Learning tools is crucial. The market offers various AI-powered solutions, from anomaly detection systems to automated threat response platforms. Choose tools that align with your organization's specific needs and security goals. Collaborating with AI experts can help select and implement the most suitable technologies.
The success of AI integration largely depends on cross-functional collaboration. Cybersecurity, IT, and data science teams must work closely to effectively implement and manage AI systems. This collaboration ensures that AI tools are correctly configured and continuously optimized.
Ongoing training and education are also essential. As AI and Machine Learning technologies evolve, staying updated with the latest advancements is crucial for maintaining an effective security posture. Regular training sessions can help staff understand how to work with AI tools and interpret their outputs.
Challenges and Best Practices in Implementing AI and Machine Learning in QA
Implementing AI and Machine Learning in QA is challenging despite the numerous benefits. One of the primary challenges is data quality. AI systems rely on large volumes of data to function effectively. Only complete or accurate data can lead to accurate results, undermining the effectiveness of the entire QA process. It's essential to ensure that data used for training and testing AI systems is comprehensive and representative of real-world scenarios.
Another challenge is the potential for algorithm biases. Machine Learning models are only as good as the data they're trained on. The AI system may produce biased outcomes if the training data contains biases. Monitoring AI systems for any signs of bias and adjusting algorithms to ensure fair and accurate results is crucial.
Another significant hurdle is the need for more AI and Machine Learning expertise. Implementing these technologies requires specialized knowledge that may be available in various ways. Organizations may need to invest in hiring or training personnel with the necessary skills or partnering with external experts.
Organizations should adhere to best practices to overcome these challenges, starting with small, manageable projects and gradually scaling up. This approach allows for the refinement of AI systems and the development of internal expertise. Additionally, staying informed about the latest AI and Machine Learning trends and incorporating new tools and techniques can help maintain a robust cybersecurity posture.
Future trends suggest that AI and Machine Learning will continue to play an increasingly significant role in cybersecurity. As these technologies evolve, they will offer even more sophisticated tools for detecting and mitigating cyber threats.
Our Final Thoughts on Transforming Cybersecurity Quality Assurance with AI & ML
Integrating AI and Machine Learning into quality assurance is revolutionizing cybersecurity. These technologies offer unparalleled capabilities in identifying vulnerabilities, automating responses, and ensuring compliance. While challenges exist, the benefits far outweigh the drawbacks, making AI and Machine Learning indispensable components of modern cybersecurity strategies. By investing in these technologies and following best practices, organizations can build more resilient defenses against ever-evolving cyber threats. Start exploring AI and Machine Learning solutions today to enhance cybersecurity measures and safeguard digital assets.